4 Essential Questions for Enterprise Leaders to Consider Before Implementing Gen AI in 2024
Kittu lays out the four essential questions enterprise leaders are grappling with while attempting to embrace Generative AI, following sit-downs with C-level executives of Fortune 500 companies in banking, retail, software, and food & beverage.
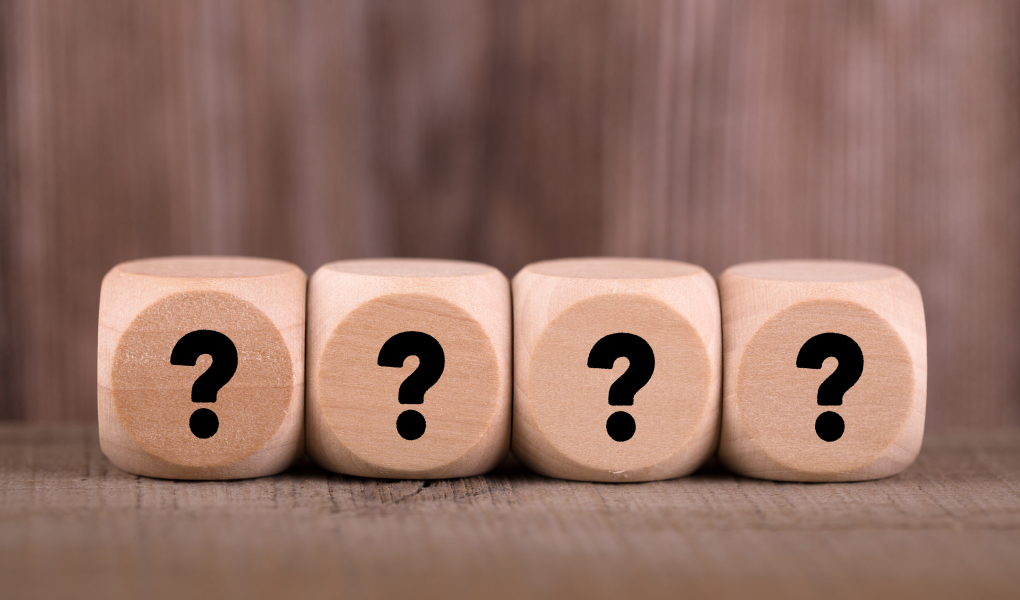
A customer logs on to the website of a global retailer. A virtual sales assistant appears and communicates so accurately, it is impossible to recognize the assistant as AI. After a few questions, the assistant generates designs for entirely new products that match the shopper’s style, fit, patterns, colors, size, and budget. The shopper clicks to buy and the products are manufactured and shipped to their doorstep. The new designs prove so popular that AI automatically generates hero images and a complete advertising campaign. The process is repeated over and over, increasing the retailer’s quarterly sales by double digits.
While this scenario is still ever-so-slightly futuristic, the requisite technology exists today. Generative AI is already changing business as we know it. To learn more about how enterprises are using generative AI and what’s in store for the remainder of 2024, I reached out to C-level executives of Fortune 500 companies in banking, retail, software, and F&B. Here’s what I learned:
Enterprise executives see the potential of Generative AI.
Conversational commerce, product design, and marketing are just some of the clear use cases for generative AI. It is also changing middle and back office functions by autonomously reviewing, synopsizing, and routing documents through enterprise workflows. Formerly tedious accounting communications are being replaced by AI that creates personalized and on-message invoices, emails, and reminders. One-size-fits-all staff training is being replaced by customized instruction according to employees’ actual experience and skills. And that is just the beginning.
The executives I spoke with shared use cases in enterprise search, demand forecasting and supply chain management, staff recruitment, software development, data analytics, tax form population, compliance and legal functions, cybersecurity penetration testing, and more. While retrieval augmented generation (RAG) implementations of generative AI are “not quite ready for prime time,” these intra-enterprise use cases have a higher tolerance for error and are therefore seen as ideal starting points for leverage of Gen AI.
They also understand the risks this technology poses.
While enterprise leaders are excited about the upside of generative AI, they are wary of implementing the technology too quickly. They worry about protecting their brands, safeguarding sensitive company information and customer data, being exposed to hacking, violating regulatory compliance, and managing unsanctioned internal use of generative AI technologies.
In my conversations, four questions emerged that executives say hold them back from going all-in on generative AI:
1. Can I trust Gen AI in customer interactions?
Large language models are a natural fit for customer conversations, but enterprises are “once bitten, twice shy” after previous AI chatbots went famously wrong. Generative AI’s infamous hallucination problem, they worry, could lead to transaction mishaps, angry customers, and brand degradation. Because generative AI feels so “human,” the bar for accuracy is high. “Don’t let AI communicate anything you wouldn’t personally put in an email now,” one exec told me. In regulated fields like banking and investment, Gen AI is considered too risky. For now, most enterprise executives are focusing on a “human in the loop” approach, viewing generative AI as a tool to help agents access information quickly and make better decisions.
2. How do I protect my IP?
A second area of concern is intellectual property status. Legal challenges by plaintiffs claiming infringement of their assets for generative AI training pose liabilities to enterprises who use these models. Meanwhile, there are limitations on protectability of AI-generated code, digital assets, and physical products. (The US copyright and patent offices have each ruled that works where AI assists humans are eligible for protection, but wholly AI-created works are not). In addition, for enterprises like insurance companies and lenders, the data that goes into actuary and lending models are a form of IP. Gen AI that is trained on this data becomes an encapsulation of those proprietary models. If someone can access that, they have everything. Instead of commingling their own data with popular generative AI offerings, enterprises prefer to bring AI to the data via smaller, open-source models, safe inside of walled gardens.
3. What new cybersecurity risks exist?
The capabilities that make generative AI a revolutionary tool for software development also create daunting new challenges. Phishing schemes and man-in-the-middle attacks are more effective when AI writes convincing text in perfect English, perhaps even in the tone of a trusted individual. Deep fake videos and voice clones take that a frightening step further, convincing employees that they are conversing with their boss or customer rather than a bad actor. Although Gen AI can be used by cyber teams to identify and patch vulnerabilities, attackers can generate endless malware assets at virtually no cost, ultimately overcoming defenses. AI code may even inadvertently create vulnerabilities that competent human coders would not. Last but not least, enterprise tech leaders have not yet solved how to authenticate access to generative AI models to ensure that only authorized staff can touch proprietary assets.
4. What about incumbent AI investments?
The last concern relates to neglecting existing AI investments. In 2022, corporations invested $93.5 billion in more mature forms of artificial intelligence. As exciting as Gen AI is, execs worry that FOMO may divert attention (and budget) from incumbent investments. “Don’t throw the baby out with the bath water,” one exec told me, “Gen AI is the flavor of the month, but other deployed and battle-tested AI technologies offer great value, with far fewer risks.” Computer vision used in retail and F&B, for instance, provides real-time customer insights and demand forecasting. AI analytics in manufacturing and operations helps reduce energy consumption, provides predictive equipment maintenance, and manages robotic process automation.
As generative AI matures, the possibilities will only expand. For now, enterprises are taking a cautious approach. As another executive described to me, generative AI today is like the “free trial version of a video game,” where you get to play just long enough to get a taste of the full experience. Hang on then—the fun has only just begun.