The Rapidly Diminishing Cost Curve: Why We May Not See a Winner Take All in Generative AI
GenAI may not follow the "hyperscalability winner takes all" effect that was seen for cloud resources. Why? The rapidly diminishing cost curve. Neeraj explores why we may in fact see a diverse cast of domain-specific competitors bubble up instead.
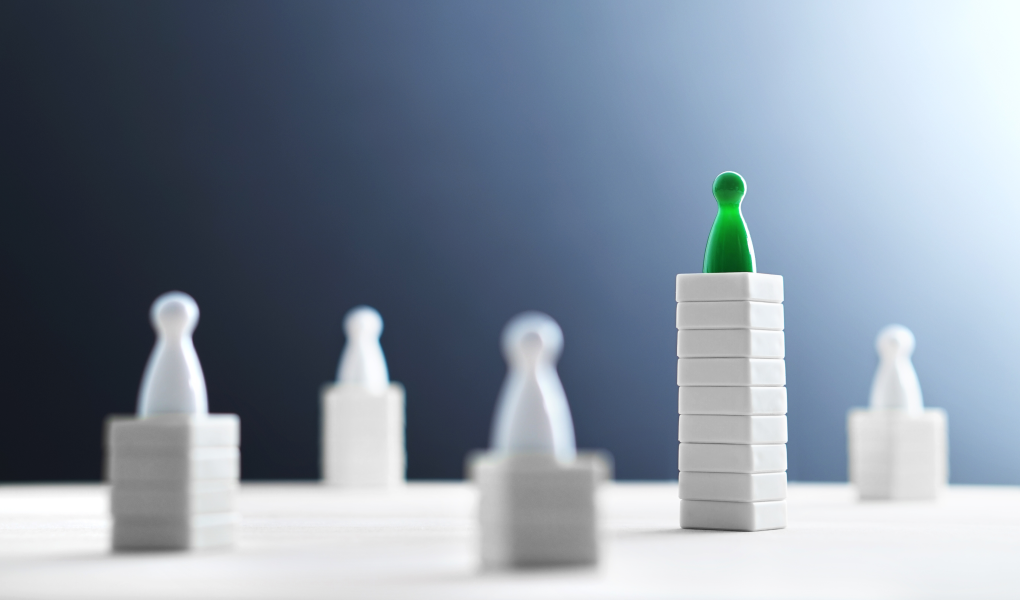
The tech players that came to dominate in the first two decades of this century had a big advantage: Computing power was expensive, but the cost of capital was next to nothing. That produced fertile ground for Microsoft, Amazon, and Google to invest a huge amount of money in achieving seemingly infinite computing power. The cost of operating their cloud-based business models on top of that infrastructure was marginal.
The capital advantage these three enjoyed created a flywheel effect that was hard to catch up with. And so these hyperscalers eclipsed other software, search, and ecommerce players so thoroughly that their brand names became metonyms for those services. The success of this approach affirmed a “hyperscaling winner takes all” precedent that the market has come to expect from AI as well.
Is GenAI following the same trend as cloud computing?
At first glance, it appears that it is. ChatGPT, already widely viewed as the “Google” of large language model (LLM) chatbots, raised $11.3 billion dollars—nearly 50% more than Anthropic, its nearest competitor—and is set to raise more, at a whopping $100 billion valuation.
Considerable cash was needed. The first GPT model cost many millions of dollars to build, and GPT-4 reportedly upped the ante to over $100 million dollars in training costs. Not only that, but OpenAI’s partnership with Microsoft gave them access to a massive amount of computing power—something that would have otherwise nearly doubled their early-stage cash requirements.
That’s a formidable competitive advantage. If the generative AI market follows the path of tech in the previous 20 years, OpenAI appears poised to leave all other players in the generative AI dust. Businesses should be wary of becoming over-reliant on any single player, and thus once again at the mercy of a behemoth the way that they are beholden today to Microsoft, Google, and Amazon for cloud resources.
Generative AI faces a rapidly diminishing cost curve.
All told, however, it is far from certain that the same rules will apply this time around. Return on investment is not fully baked into Open AI’s pricing yet. GPT+ subscriptions are running at just $20/month and professional user token prices have repeatedly declined. ChatGPT faces real challengers in Meta’s LLama, Google’s Bard, and Anthropic’s Claude, while Dall-E competes with Stable Diffusion, Midjourney, and others. The war for dominance in generative AI is far from over.
But there is something brewing that may be an even greater threat to OpenAI’s hegemony than its largest competitors:
What if generative AI development no longer requires that much capital? What if the cost curve rapidly diminishes to the point where anyone can join in? Were that to happen, we could end up with a whole cast of small competitors, niche markets, and home-grown enterprise solutions, rather than just two or three monolithic players. There are three ways this might happen:
- More accurate data:
For domain-specific use cases in the enterprise, generative AI models can produce more accurate outcomes when given access to “the right data” rather than “the most data.” Retrieval-augmented generation (RAG) allows users to supplement existing LLM models with documents from an enterprise’s own knowledge base such as product sales literature, customer service tickets, and technical manuals. This produces far more accurate outcomes and mitigates the hallucination problem of heavy-duty LLM models. It also allows users to deploy lighter-duty, lower-fee LLM models like ChatGPT 3.5 Turbo, resulting in a “staggeringly low” cost.
Enterprises do not even need to build this integration themselves. Neotribe portfolio company IrisAgent, for instance, trains its customer service chatbots on the existing knowledge base of its enterprise client companies. These chatbots are able to analyze customer sentiment, communicate organically, and solve problems accurately, with far less computing power than the largest LLM models on the market. This approach augurs a rapid deceleration of the generative AI cost curve.
- More efficient processing:
Where large amounts of data are required, some generative AI players are already working on achieving more performance with less computing power. ChatGPT’s infrastructure is built with tens of thousands of NVIDIA’s most advanced enterprise-grade graphics processing cards (GPUs), like the A100 and H100, which cost hundreds of millions of dollars. No small startup can compete with that.
But what if you didn’t need such firepower? What if, instead of using a $10,000 GPU, you could use an abundantly available central processing unit (CPU) that costs just a few hundred dollars? That would unlock the ability to compute on-premises at close to zero marginal cost, instead of paying for third-party processing in the cloud. And, at least with relatively small generative AI models, you already can. Neotribe portfolio company ThirdAI’s BOLT engine is capable of training multi-billion parameter LLM models and processing billions of tokens per day—faster than a NVIDIA A100—using only a CPU.
This not only enables enterprises to develop bespoke generative search products using their own vast collections of documents, but also empowers anyone to create a purpose-built LLM model using only a consumer-grade laptop. More efficient generative AI training and processing means that enterprises need not remain beholden to large players like OpenAI for compute. That may make it difficult for a singular approach to dominate the market.
- Enterprise preference for walled gardens:
A third reason that there may never be one generative AI player “to rule them all” is the need of enterprises for control and transparency. Many sectors—finance and healthcare, for instance—have regulatory restrictions, bureaucratic burden, and other incentives to carefully safeguard their data. While OpenAI’s enterprise privacy policy says that customers own and control their own data and that they will not train their models using customer data, enterprises still may not be that comfortable sending their data off to the cloud to be processed.
Corporate users may prefer the DIY approach for its benefits of customization. Purpose-built AI may convey more accuracy and can be reinforced to produce the kind of output that a single enterprise requires. Proprietary models offer better control over intellectual property, while we wait to see how the law will regard AI-generated images, text, code, and other IP. Purpose-built generative AI may therefore come to be seen as a key competitive advantage against players using off-the-shelf, closed-source models.
For all of those reasons, enterprises may opt to develop their own solutions, or use smaller, more portable models that can live on their own servers, instead of third-party services that do not allow them total transparency and control.
It seems far from certain that “those with the gold will make the rules,” this time around. It’s quite possible that we will instead see a diverse cast of domain-specific competitors bubble up, just like in the general software market. Whether that happens or not, it is clear that the moat for Open AI and its prime competitors is not nearly as deep as it was for Google, Amazon, and Microsoft in the cloud wars. With the stakes as high as they are in AI, I expect that startups, investors, and enterprise buyers will continue to keep a close eye on the concepts and technologies that hold the potential to rapidly diminish the generative AI cost curve.